How Will Self-Flying Aircraft Make Ethical Choices?
Experts weigh in on how electric vertical takeoff and landing (eVTOL) air taxis will fly autonomously and how aircraft controlled by artificial intelligence/machine learning systems might respond during life-and-death situations.
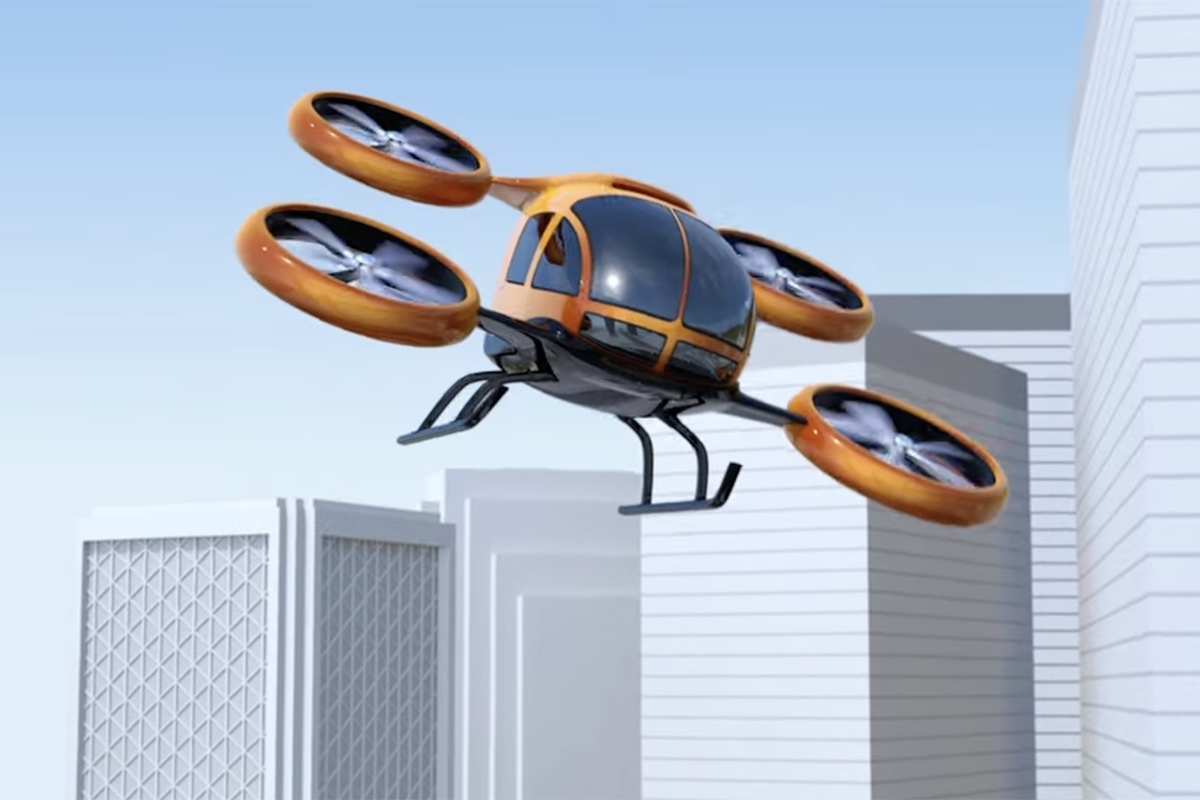
Perhaps as soon as 2035, aircraft piloted by artificial intelligence/machine learning (AI/ML) systems will have to perform aeronautical decision-making functions—and make ethical choices previously reserved only for humans. [Courtesy: EASA]
It’s no secret that many companies developing electric, vertical takeoff and landing (eVTOL) air taxis eventually plan to fly their fleets autonomously—with no pilot on board to guide aeronautical decision making (ADM). Eventually, experts believe the machines that will pilot these aircraft will be capable of learning and making difficult ethical choices previously reserved only for humans.
For example, let’s imagine the year is 2040. You’re a passenger in a small, autonomous, battery-powered air taxi with no pilot flying about 3,000 feet over Los Angeles at 125 mph. Air traffic is crowded with hundreds of other small, electric aircraft, flying and electronically coordinating with each other, allowing very little separation. Suddenly, an alarm goes off, warning about another passenger aircraft on a collision course. But amid heavy traffic, there are no safe options to change course and avoid the oncoming aircraft. In this scenario, how would a machine pilot know what action to take next?
For engineers and ethicists, this scenario is commonly known as a “trolley problem,” an ethics thought experiment involving a fictional runaway trolley, with no brakes, heading toward a switch on the tracks. On one track, beyond the switch, are five people who will be killed unless the trolley changes tracks. On the switched track is one person who would be killed. You must decide whether to pull a lever and switch tracks. Should you stay the course and contribute to the deaths of five people or switch tracks, resulting in the death of just one?
The analogy is often used in teaching ADM. Someday, aircraft controlled by artificial intelligence/machine learning (AI/ML) systems may face a similar quandary.
“I don’t want to trivialize this problem away, but we should not have a system that has to choose every time it lands, who to kill,” says Luuk van Dijk, founder and CEO of Daedalean AG, a Swiss company developing flight control systems for autonomous eVTOLs.
Van Dijk says even human pilots facing trolley problems rarely have the luxury of making a balanced choice between two bad options.
“I think we can design systems that can deal with that kind of variation in the environment and figure out on the spot, even before the emergency happens,” says van Dijk, whose resume includes software engineering positions at Google and SpaceX.
“This is another advantage of the machine. It can have a plan ready if something were to happen now, rather than figuring out that there is something wrong and then going through a checklist and coming up with options,” he says. “If you want [machines] to truly fly like humans fly, you have to make robots that can deal with uncertainty in the environment.”
Currently, software developers and aeronautical engineers have gotten very good at programming machines to pilot aircraft so they can safely and reliably complete repetitive tasks within predetermined parameters.
But as the technology stands now, these machines still can’t be creative. They can’t come up with instant, creative solutions to unanticipated problems that may suddenly threaten aircraft and passengers. They can’t teach themselves to get out of extremely unfortunate and unpredictable coincidences based on previous flight experience.
That’s the promise of AI/ML—often called the holy grail of automated aviation.
Learning Machines in the Left Seat
So, what are AI/ML systems, exactly? According to the European Union Aviation Safety Agency’s (EASA) Artificial Intelligence Roadmap, they use “data to train algorithms to improve their performance.” Ideally, EASA says, they would bring a computer’s “learning capability a bit closer to the function of a human brain.”
Van Dijk says the term artificial intelligence is “really very badly defined. It’s a marketing term. It’s everything we don’t quite know how to do yet. So by definition, the things we don’t quite know how to do yet are uncertifiable because we don’t even know what they are. When people talk about artificial intelligence, what they mostly mean is machine learning.”
“The systems that we do—and what you might call artificial intelligence—use a class of techniques that are based on statistics and try to handle this amount of uncertainty.”
Statistical AI is data-driven. In the case of aviation, the machine pilot would control the aircraft based on a constant stream of data, gathered by multiple inputs from onboard cameras, radar, Lidar, and other sensor equipment—combined with live, real-time data from a central air traffic control system. The machine pilot also might be supported by a massive GPS database of existing buildings and infrastructure on the ground, relevant to a predetermined flight path.
The first iterations of these automated pilot systems would rely on sophisticated detect-and-avoid (DAA) systems. The machine pilot would monitor sensor data that detects potential obstacles and quickly command the aircraft’s flight control systems to change course when needed to avoid the obstacles. Despite award-winning innovations such as Garmin’s Autoland, the aviation industry is still a long way from truly automated aircraft that can anticipate and then react safely and responsibly to infinite numbers of possible combinations of unpredictable events. Remember, a human still has to turn Autoland on.
‘Mysterious Neural Net’
Early automated eVTOLs will not include a “mysterious, neural net—a complex brain in a black box that tries to watch other aircraft and learn from them,” says Wisk Aero’s head of autonomy, Jonathan Lovegren. Wisk’s piloting system will be based on “a foundational, deterministic, rule-based approach to flight—akin to a Boeing 737 that’s on autopilot for most of the flight. It’s largely the same process and approach as developing and certifying an autopilot system.” A key point to keep in mind here is that a 737—or any commercial airliner with autopilot—has two human pilots onboard who can apply ADM to offset any unwanted actions by the autopilot.
Based in Mountain View, California, Wisk Aero was started by Google co-founder Larry Page. The privately held company has been flight testing eVTOLs for years and recently received a $450 million investment from Boeing. Its fifth-generation, automated, two-passenger air taxi named Cora has been flying since 2018. The company has been quiet about its expected timeframe for certification and entering service, saying it’s focusing on safety first.
Lovegren and his colleagues are including FAA pilot training standards as guidelines for programming Wisk’s air taxis, “to make sure there’s no gap between all the functions performed by a pilot and in the system that we’re building. It’s actually much more foundational and rule based … fundamental aerospace engineering, using math.”
Although Wisk is calling its aircraft design “autonomous,” it can’t truly be autonomous until it applies to each flight past learning from disparate sources outside the flight. That will be a big challenge to solve.
Nonetheless, it is “very highly automated,” Lovegren says. It has to make some decisions on its own, and a large engineering effort is required to determine what those responses are. “It’s not like it’s making decisions and nobody knows what it’s going to do.”
What about ethical piloting decisions? Wisk automated air taxis will always have “an operator who is going to be on the ground,” Lovegren says. The human component of Wisk air taxis will be “much more like an air traffic control type of interface,” he says. “The aircraft can make immediate decisions in the name of safety—dealing with failures and whatnot—but largely, there’s a person in the loop monitoring the flight and directing where it’s going.”
Wisk is applying a large foundation of existing DAA technology to its flight control system, Lovegren says, such as TCAS II and the upcoming ACAS. He sees both as stepping stones that are leading to automated responses to DAA problems.
‘Major Ethical Questions’
Aviation regulators have begun making plans to integrate AI/ML into commercial aviation in the next few decades, although that’s no guarantee it will become a reality.
The FAA has completed an AI/ML research plan that will help the agency begin to map out the certification process. Similar efforts are underway in Europe, where EASA is projecting commercial air transport operations will be using AI/ML as soon as 2035.
In its “Artificial Intelligence Roadmap,” EASA acknowledges that “more than any technological fundamental evolutions so far, AI raises major ethical questions.” Creating an approach to handling AI “is central to strengthening citizens’ trust.”
EASA says AI will never be trustworthy unless it is “developed and used in a way that respects widely shared ethical values.” As a result, EASA says “ethical guidelines are needed for aviation to move forward with AI.” These guidelines, EASA says, should build on the existing aviation regulatory framework.
Ethical Guidelines
But where are these “ethical guidelines” and who would write them?
Well, it just so happens that an outfit calling itself the High-Level Expert Group on Artificial Intelligence wrote a report at the behest of the European Commission called, “Ethics Guidelines for Trustworthy AI.”
The non-binding report says its guidelines “seek to make ethics a core pillar for developing a unique approach to AI” because “the use of AI systems in our society raises several ethical challenges” including “decision-making capabilities and safety.”
It calls for “a high level of accuracy,” which is “especially crucial in situations where the AI system directly affects human lives.”
What if my AI pilot screws up? Well, in that case the report suggests systems “should have safeguards that enable a fallback plan, including asking “for a human operator before continuing their action. It must be ensured that the system will do what it is supposed to do without harming living beings or the environment,” the report says.
Who should be responsible for AI systems that fail? According to the report, “companies are responsible for identifying the impact of their AI systems from the very start. It suggests ways to ensure AI systems have been “tested and developed with security and safety considerations in mind.” The report also proposes the creation of ethics review boards inside companies that develop AI systems to discuss accountability and ethics practices.
The report also says AI systems should be “developed with a preventative approach to risks…minimizing unintentional and unexpected harm, and preventing unacceptable harm.”
Why is eVTOL Focusing on Automation?
The short answer is: affordability and safety. Proponents say autonomous flight will allow eVTOL airlines to more effectively and quickly scale up, while driving down fares—ideally—making eVTOL transportation available to more people.
Putting machines in the left seat, so to speak, will make eVTOL flights safer, according Wisk and others, who say automated flight reduces the potential for human error.
Statistics blame most aviation accidents on pilot error. Wisk and other eVTOL developers say autonomous systems can dramatically reduce human errors by creating predictable, consistent outcomes with every flight. What remains to be seen is to what degree machine error would replace human error. Countless pilots have experienced automation that executes an unexpected command—or outright fails.
Self-Flying Aircraft vs. Self-Driving Cars
Self-flying aircraft engineers agree that their task would certainly be even more challenging if they were trying to perfect self-driving cars.
“I do not envy the people who are working on self-driving cars,” Lovegren says. “It’s much easier in a sense to operate in the airspace than it is to operate on the ground when you could have a kid running out with a soccer ball in the middle of the street. How do you deal with that problem?” In the air, “you’re operating with professionals, largely. I think it makes the scope of the autonomy challenge much more manageable, certainly in aviation.”
Van Dijk agrees: “Driving is much harder.” It would be much more difficult to build a system that has to read road signs and be able to understand the difference between a rock and a dog and a pedestrian, he says. In a car, you have limited options for changing course. “The situation where you have to choose who to kill arises more naturally in a driving situation than in the air,” van Dijk says. “In the air, the system can be taught to avoid anything you can see, unless you’re really sure you want to land on it.”
‘Ethical Ordering’
Computer programming engineers often talk about how a “rational agent” must be part of an autonomous aircraft’s decision-making process. The aircraft must be smart enough to know when to turn off its autopilot and command the aircraft in the safest, most ethical way possible.
To do that, a so-called “ethical ordering” of high-level priorities must be programmed into the system, according to University of Manchester computer science professor Michael Fisher.
Fisher offers a very simple example involving a malfunctioning autonomous aircraft with limited flight controls. Ideally, the aircraft’s rational agent must have the wherewithal to realize it must disengage autopilot and make an immediate emergency landing. In Fisher’s example, the rational agent controlling the aircraft has three choices for landing locations:
- a parking lot next to a school full of children
- a field full of animals
- an empty road
This scenario would trigger the rational agent to refer to a pre-programmed ethical ordering of high-level priorities in this order:
- Save human life
- Save animal life
- Save property
As a result, the autonomous aircraft would attempt an emergency landing on the empty road. Of course this is an overly simple scenario. Any real-world situations similar to this would require sophisticated ADM programming that would instruct the flight control system how to react to various iterations of this scenario, such as a school bus that might suddenly appear on the road.
With such extremely limited options, would an AI/ML system be smart enough to solve that problem?
Merging AI/ML with Air Traffic Control
While the FAA is developing a roadmap for integrating certification requirements for AI/ML systems, NASA’s Ames Research Center in Mountain View, California, has been conducting long term research to learn more about how AI/ML autonomous aircraft might eventually be integrated into the national airspace.
Dr. Parimal Kopardekar, director of NASA’s Aeronautics Research Institute (NARI) at Ames, believes digital twinning will play a key role.
Here’s how it would work: Once the AI/ML systems are developed, engineers might create digital models that mimic the systems in a virtual airspace. This virtual airspace would mirror a real airspace, and show performance in real time.
Engineers would use this digital twin experiment to gather cloud-based data on how the AI/ML aircraft perform in real-world scenarios, with no risk. Successful data collected over a long period of time could provide enough assurance that the systems are safe and reliable enough for deployment in the real world.
“We want autonomous systems to be trustworthy, so people can feel that they can use it without hesitation,” Kopardekar says. “We want them to be fully safe.”
Although the commercial aviation industry is currently experiencing the safest period in its history, we know that no aviation system will ever be truly foolproof and immune to accidents. Engineers and programmers are painting an optimistic picture about the challenges of developing successful AI/ML flight control systems, including associated ethical questions.


Sign-up for newsletters & special offers!
Get the latest FLYING stories & special offers delivered directly to your inbox